Guest post by Julia Ligteringen, Business Development and Customer Success Manager at Leximancer
Guest posts express the views of guest contributors and do not necessarily represent the views of the CAQDAS Networking Project or constitute an endorsement of any product, method or opinion
You’re staring at your screen, enthralled by a perfectly coherent response from an AI tool. It’s elegant, polite, and speaks with the authority of a seasoned expert. The words flow so smoothly that you don’t pause to question them. Why would you? It sounds reasonable—even irrefutable. Yet, beneath the charm lies a flaw that should not be overlooked: AI doesn’t understand a single word it just said.
This phenomenon isn’t new. It’s rooted in our cognitive biases, the way we’re wired to trust voices that sound knowledgeable and confident. Large language models (LLMs) tap into this psychological vulnerability, drawing us in with their veneer of expertise. But why are we so drawn to this illusion of intelligence? And what does it mean for the way we interact with these systems?
The Allure of a Polished UI
The user interfaces of LLMs are a masterclass in persuasion. Minimalist, intuitive, and reassuringly efficient, they invite users to dive in without hesitation. These interfaces are not unlike the carefully curated feeds of social media platforms. Just as algorithms on Instagram or Facebook are designed to keep us scrolling, LLMs subtly encourage us to keep engaging, asking more questions, and feeding it more prompts.
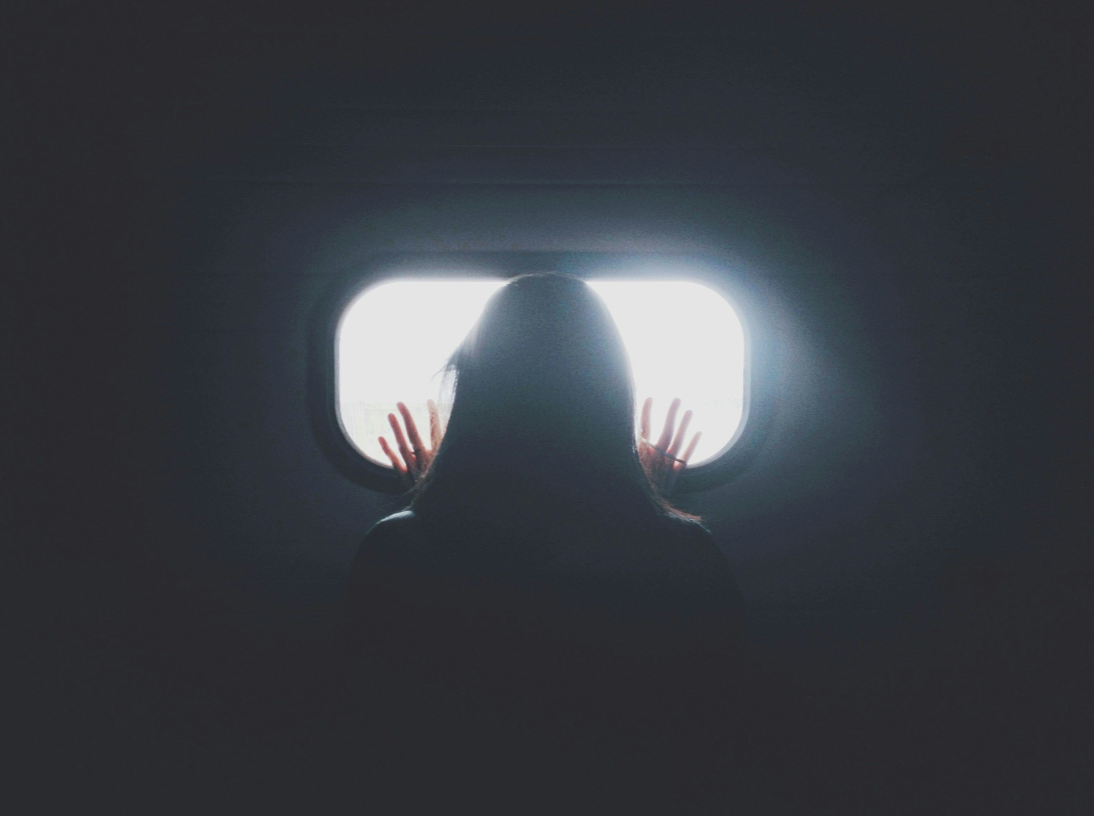
The appeal goes beyond design. LLMs speak in a tone that’s firm yet polite, striking the perfect balance between authority and approachability. This first-person appeal feels personal, almost human. It’s no wonder we’re captivated.
The terror of hallucination: so slick, so glib, so confident—it must be true.
But why are these tools designed this way? The answer lies in their underlying business model. Large AI platforms are owned by billionaires and corporations whose primary goal, as always, is to maximise profit. It’s a cycle of dependency: the more prompts you input, the more reliant you become on these tools, and the more the corporations behind them profit. This design isn’t just about offering a useful tool; it’s about ensuring you keep coming back.
The Danger of Confidence Without Understanding
Here’s where things get tricky: the AI’s confidence is often misplaced. It glues together two distinct pieces of regurgitated information that don’t belong in the same sentence, creating what’s called “hallucinations” (a fancy word for lies). And yet, the delivery is so polished that we rarely notice the cracks.
This poses a critical question for us as users: is it okay to rely on an AI trained on vast but imperfect datasets? When it answers, it doesn’t reason or analyse; it retrieves patterns it has seen before. If the data is flawed or biased, so too are the responses. And yet, because the words are arranged so convincingly, we often accept them without question.
You can imagine the dangers this invites.
If more people are using LLMs and accepting hallucinations as truth – this creates a new breed of misinformation. The lie becomes truth as it’s reabsorbed back into the model as fact. As AI content becomes increasingly ubiquitous, people unknowingly absorb falsehoods. A well-written lie can slip past even seasoned professionals. Over time, as misinformation compounds, it risks reshaping public understanding, eroding trust in credible sources, and leading us back into an age where truth is an elusive concept.
Why We Embrace Cognitive Bias
Our susceptibility to cognitive bias plays a significant role here. We’re drawn to things that confirm our existing beliefs or feel familiar. When an AI echoes back our ideas, we’re inclined to trust it. This bias isn’t inherently bad—it’s a natural part of how humans process information. But when interacting with AI, it can blind us to its limitations.
Asking the Right Questions
To break free from this trap, we need to approach AI with a critical mindset. The key is to ask the right questions:
- Is it appropriate to use this training set for this particular task?
- What assumptions am I making about the AI’s capabilities?
- How can I ensure that the outputs align with the truth, not just a convincing narrative?
These questions are essential for responsible AI use. And they’re especially critical for those of us using tools for tasks like qualitative analysis, where understanding the nuances of language is paramount.
How Tools Can Help Without Trapping Us
This is where thoughtful technology can make a difference. For instance, Platforms like Leximancer, and other tools used in qualitative data analysis, don’t rely on pre-existing coding schemes or the illusion of understanding. Leximancer works by giving words statistical values and mapping their relationships to identify and visualise concepts, offering a rigorous and unbiased foundation for interpretation. By prioritising transparency and reproducibility, these tools empower researchers to critically engage with their data rather than simply accepting polished guesses.
In contrast, some generative AIs attempt to compensate for the limitations of LLMs by locating external information to guide their outputs with what is known as Retrieval-Augmented Generation, or RAG. While this approach may appear to offer more accuracy, it still relies on external sources that may be flawed, biased, or outdated. The integration of retrieved data into the model’s response often lacks transparency, leaving users unable to assess the reliability of the outputs fully. You can read more on this subject here: Everything Wrong with Retrieval- Augmented Generation
And so the distinction becomes clear. While LLMs can provide quick insights, tools designed with rigour and specificity in mind help us step back and analyse with clarity. They encourage us to become experts in our topics, to dig deeper into the reasoning behind the data, and to resist the allure of glib, overconfident narratives.
A Call for Awareness
There is no doubt we will continue to further integrate AI into our lives. But by doing so, know it’s essential to stay mindful of its limitations. The shiny halo effect of LLMs may be appealing, but we mustn’t mistake it for understanding. Instead, let’s strive for a balance: embracing the convenience of AI while maintaining the critical thinking that makes us uniquely human.
After all, good work—the kind we can be proud of—requires more than just a smooth surface. It demands depth, nuance, and a willingness to question the lie, no matter how convincing it may seem…. or how inconvenient.
AI use: 95% Julia, 5% Sam Altman