Philip Aston attended an IPEM (Institute of Physics and Engineering in Medicine) workshop on The Effect of Skin Pigmentation on Pulse Oximeter Accuracy in London on 22nd May 2024. He gave a talk entitled “Does skin tone affect the accuracy of machine learning classification applied to photoplethysmography signals?”.
During the pandemic, it became apparent that pulse oximeters were giving inaccurate readings of blood oxygenation for patients with darker skin, which could potentially lead to harm, and so an independent review was established to look into this and other issues. The report Equity in Medical Devices was published in March 2024. Smart watches work in the same way as pulse oximeters, so the photoplethysmography (PPG) signal that they generate is also likely to be affected by skin tone. PPG signals are being increasingly used with machine learning to extract other physiological parameters of interest, for example blood pressure. Philip and his team therefore addressed the question of whether skin tone affects the accuracy of machine learning. They chose a simple binary classification problem to classify blood pressure as “high” or “not high”. They trained a machine learning model using only subjects with Fitzpatrick Skin tone 1 (lightest skin tone) and then tested the model using data from all the other skin tones 2-6. It was found that classification accuracy (see figure below) was reduced for all other skin tones, with over a 30% drop in accuracy for the darkest skin tone! When using all the skin tones in the training data, the best performance was again for Fitzpatrick skin tone 1 but in this case, the reduction in accuracy for the darkest skin tones was only 3%. Thus, it was concluded that it is essential that signals associated with all skin tones are included in the training data for machine learning models.
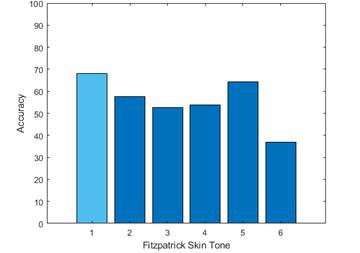