The paper “Matrix-Model Simulations Using Quantum Computing, Deep Learning, and Lattice Monte Carlo“, co-authored by Enrico Rinaldi (Michigan & RIKEN Japan), Xizhi Han (Stanford), Mohammad Hassan (City College NY), Yuan Feng (RIKEN Japan), Franco Nori (MIchigan & RIKEN Japan), Michael McGuigan (Brookhaven NY), and Masanori Hanada has been published in PRX Quantum. It is open access and the link is here. In the paper, they investigated whether new methods such as classical-quantum hybrid algorithm or variational methods, inspired by machine learning, could be used to study matrix models which are important in superstring theory. To check the results obtained by using such methods, they did independent computation using traditional lattice Monte Carlo methods. The authors concluded that the new methods are promising and hopefully they can be used to tackle a class of problems which are out of reach with traditional methods. The image below is Figure 19 from the paper showing a comparison of convergence results.
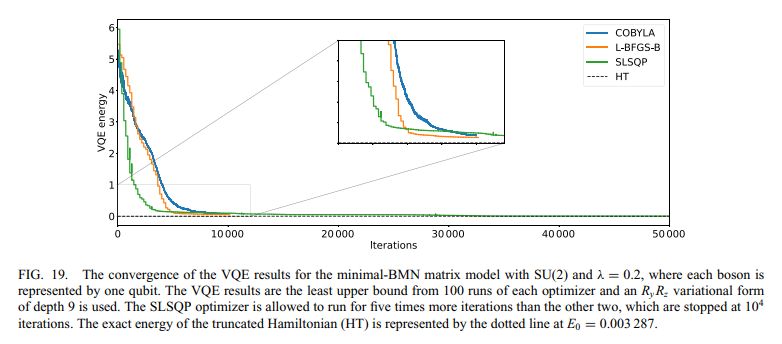